There are many outstanding questions in healthcare and medicine that Artificial Intelligence could help address. These are some important ones:
- Basic Science and Research
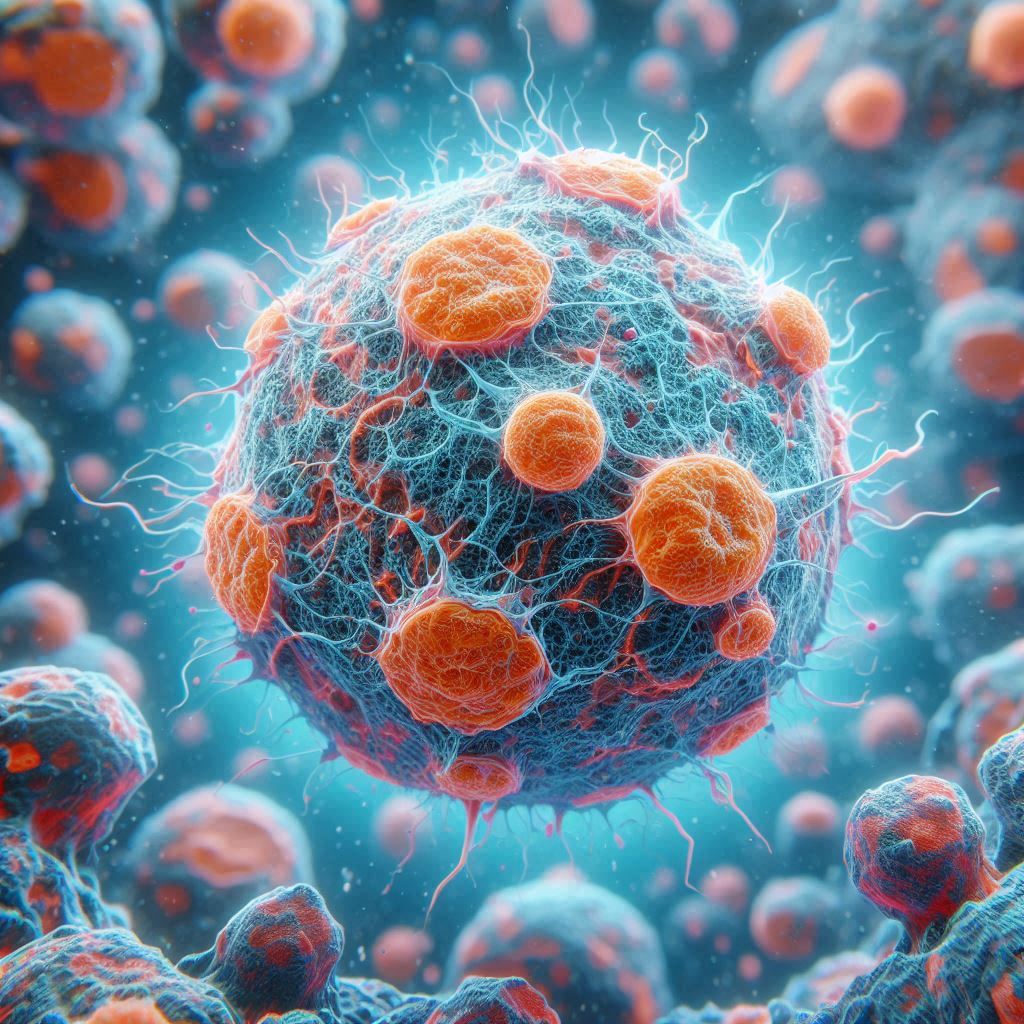
Cancer Biology and Therapy: How can we effectively target cancer stem cells to prevent relapse?
AI agents hold immense potential in the fight against cancer relapse, particularly when it comes to targeting cancer stem cells (CSCs). Here’s how AI can play a crucial role:
1. Identifying and Characterizing CSCs:
- Image Analysis: AI agents can analyze vast datasets of high-resolution images from tumor biopsies. Machine learning algorithms can be trained to identify CSCs based on specific morphological or phenotypic characteristics, aiding in their differentiation from regular cancer cells.
- Genetic and Transcriptional Data Analysis: AI agents can analyze large-scale genetic and transcriptomic datasets to identify genes and pathways specifically associated with CSCs. This helps understand the underlying mechanisms that drive stemness and treatment resistance.
2. Developing Personalized Treatment Strategies:
- Patient Data Integration: AI agents can integrate patient data, including medical history, tumor characteristics, and genetic profiles, to predict their risk of relapse and identify the presence of CSCs.
- Predictive Modeling: AI agents can be used to predict how different therapeutic approaches might target CSCs in a specific patient’s tumor. This allows for personalized treatment plans that are more likely to eradicate CSCs and prevent relapse.
3. Designing New Therapeutic Strategies:
- Virtual Drug Discovery: AI agents can be used for virtual drug discovery, simulating the interaction of potential drug candidates with CSCs at a molecular level. This can accelerate the identification of drugs that target CSCs more effectively.
- Drug Combination Optimization: AI agents can analyze drug-drug interaction data to identify synergistic combinations that target both CSCs and the bulk tumor population, minimizing the risk of resistance development.
4. Optimizing Treatment Delivery:
- Radiation Therapy Planning: AI agents can be used to optimize radiation therapy plans to deliver targeted doses to areas with a higher concentration of CSCs, while minimizing damage to healthy tissue.
- Nanoparticle Design: AI agents can help design nanoparticles that specifically target and deliver therapeutic agents to CSCs, improving treatment efficacy.
Cancer Biology and Therapy: What are the mechanisms underlying resistance to chemotherapy and targeted therapies?
AI agents are revolutionizing our understanding of cancer biology and therapy, particularly regarding the mechanisms behind resistance to chemotherapy and targeted therapies. Here’s how AI can be instrumental:
1. Analyzing Large-Scale Datasets:
- Genetic and Omic Data Analysis: AI agents can analyze massive datasets of genetic mutations, gene expression profiles, and other omics data from tumor samples. This allows for identifying patterns and correlations associated with resistance to specific therapies.
- Drug-Response Databases: AI agents can analyze large databases of patient responses to different cancer therapies. This helps identify common genetic alterations or signaling pathways associated with drug resistance.
2. Modeling Resistance Mechanisms:
- Machine Learning for Mechanism Discovery: AI agents can be used to develop machine learning models that predict how specific mutations or gene expression changes might contribute to resistance to different therapies. These models can help identify novel resistance mechanisms.
- Simulating Tumor Evolution: AI agents can be used to simulate the evolutionary dynamics of tumors under selective pressure from chemotherapy or targeted therapies. This helps understand how tumors develop resistance mechanisms over time.
3. Drug Target Identification and Validation:
- Virtual Screening: AI agents can perform virtual screening of vast chemical libraries to identify potential drug candidates that might target newly discovered resistance mechanisms.
- Synthetic Lethality Prediction: AI agents can predict synthetic lethal interactions between drug targets and resistance pathways. Targeting these interactions can overcome resistance and improve treatment efficacy.
4. Developing Personalized Treatment Strategies:
- Patient Stratification: AI agents can analyze a patient’s tumor profile to predict their likelihood of responding to specific therapies, considering their unique genetic makeup and potential resistance mechanisms.
- Combination Therapy Optimization: AI agents can analyze drug-drug interaction data to identify synergistic combinations that can overcome resistance pathways and improve treatment outcomes.
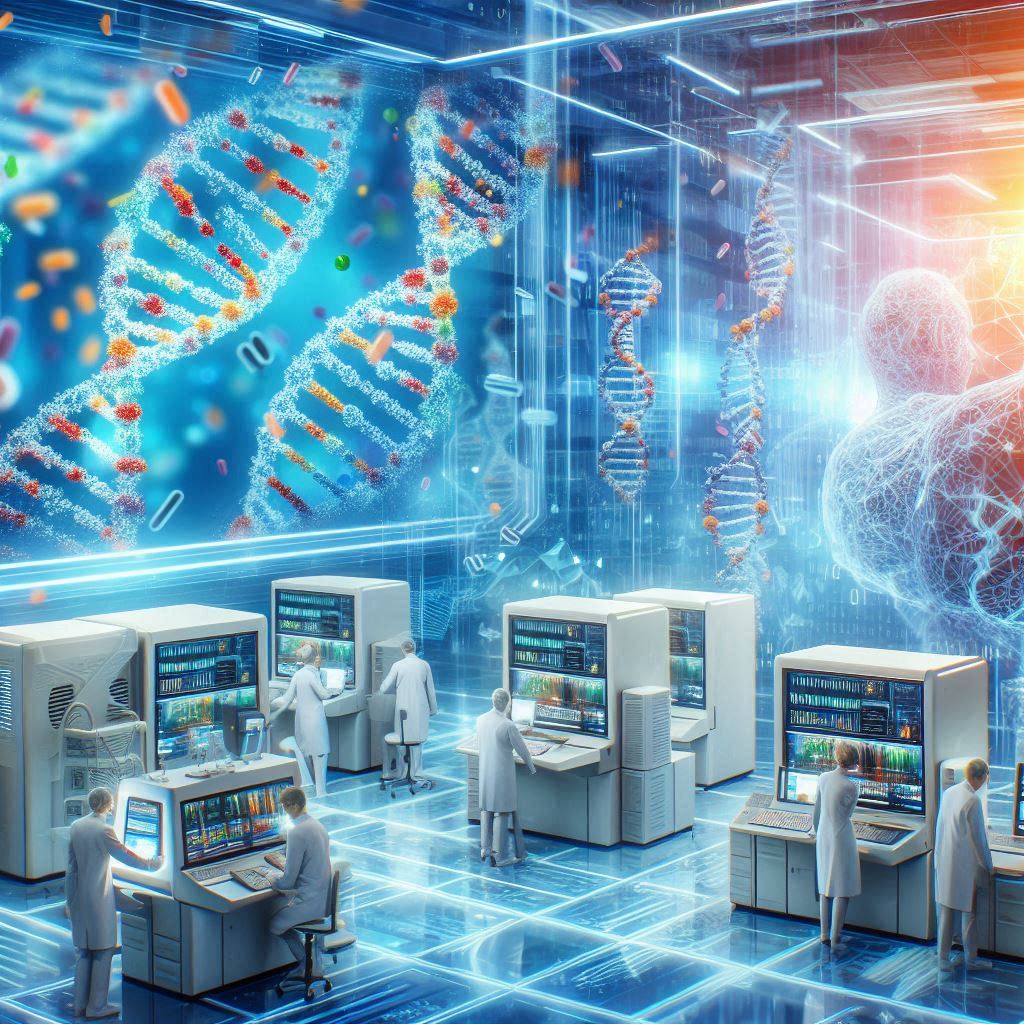
Genomics and Personalized Medicine: How can we integrate genetic, epigenetic, and environmental factors to predict disease risk and personalize treatment?
AI agents are revolutionizing personalized medicine by enabling the integration of vast amounts of genetic, epigenetic, and environmental data to predict disease risk and tailor treatment plans. Here’s how:
1. Data Analysis and Integration:
- Multi-Omic Data Integration: AI agents can analyze and integrate data from various sources, including genomics (DNA mutations), epigenomics (gene expression patterns), and environmental exposures. This allows for a holistic understanding of individual disease susceptibility.
- Machine Learning for Risk Prediction: AI agents algorithms can be trained on large datasets to identify complex interactions between genetic variations, epigenetic modifications, and environmental factors that contribute to disease risk.
2. Personalized Treatment Recommendations:
- Drug Response Prediction: AI agents can analyze a patient’s genetic and epigenetic profile to predict how they might respond to specific medications. This allows for personalized treatment recommendations with potentially fewer side effects and higher efficacy.
- Tailoring Treatment Doses: AI agents can analyze genetic and environmental factors to determine the optimal dosage of medications for individual patients, minimizing the risk of over- or under-treatment.
3. Early Disease Detection:
- Identifying High-Risk Individuals: AI agents can analyze genetic and environmental data to identify individuals with a higher risk of developing certain diseases, allowing for early intervention and preventive measures.
- Non-invasive Biomarker Discovery: AI agents can analyze data from blood tests or imaging studies to identify non-invasive biomarkers that indicate early signs of disease, facilitating early diagnosis and personalized treatment.
4. Clinical Trial Design and Patient Stratification:
- Matching Patients to Trials: AI agents can analyze patient data to identify individuals who are most likely to benefit from participation in specific clinical trials, improving the efficiency and effectiveness of clinical research.
- Subgroup Identification: AI agents can help identify subgroups of patients with similar genetic, epigenetic, and environmental profiles, allowing for more targeted clinical trials and treatment approaches.
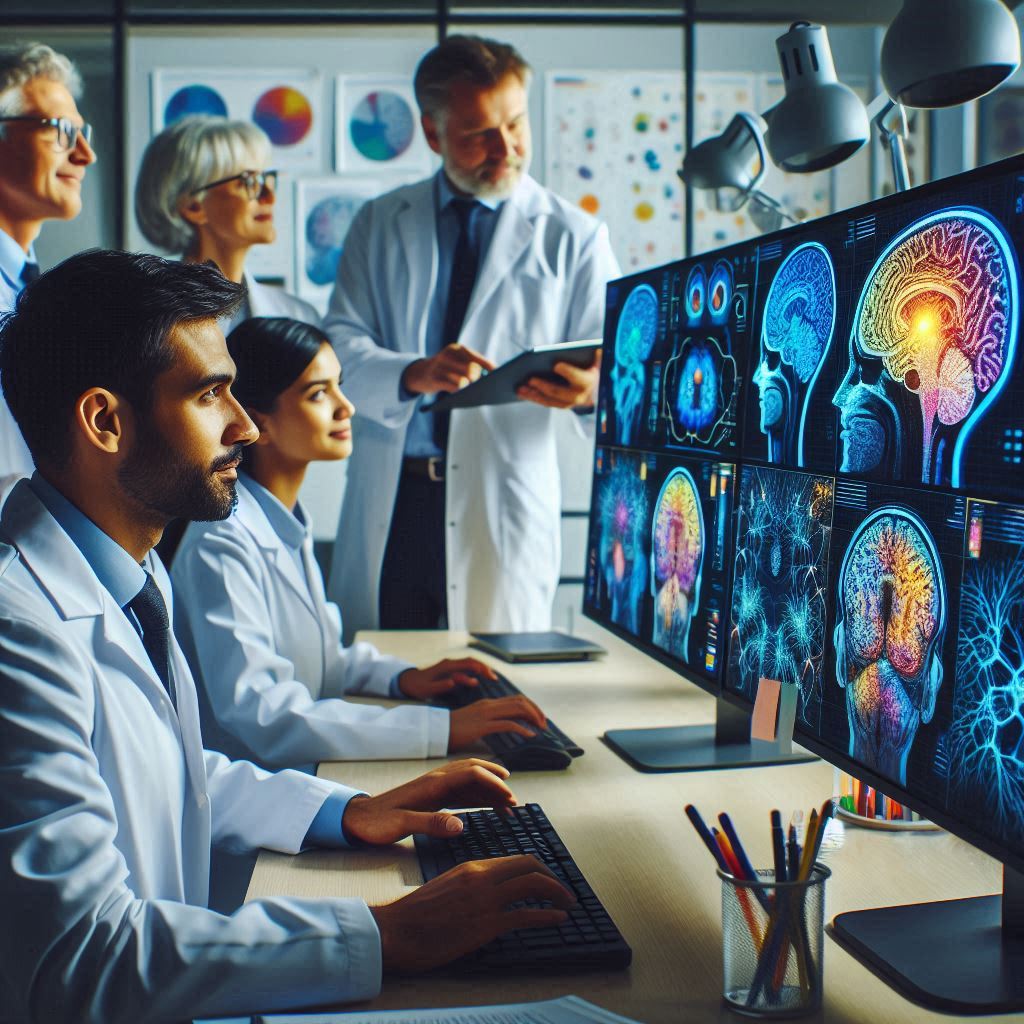
Neuroscience: What are the mechanisms underlying neurodevelopmental disorders such as autism spectrum disorder?
AI agents are emerging as powerful tools in neuroscience, aiding researchers in unraveling the complex mechanisms underlying neurodevelopmental disorders like Autism Spectrum Disorder (ASD). Here’s how AI can contribute:
1. Analyzing Large-Scale Brain Imaging Data:
- MRI and EEG Analysis: AI agents can analyze vast amounts of data from Magnetic Resonance Imaging (MRI) and Electroencephalograms (EEG) to identify subtle differences in brain structure and function in individuals with ASD compared to neurotypical individuals. These differences might provide clues about the underlying mechanisms of the disorder.
- Identifying Biomarkers: AI agents algorithms can be used to identify potential biomarkers of ASD in brain imaging data. These could be specific patterns of brain activity or structural abnormalities that could aid in earlier diagnosis and development of targeted interventions.
2. Genetic Data Analysis and Variant Discovery:
- Genome-Wide Association Studies (GWAS): AI agents can be used to analyze large-scale GWAS data to identify genetic variants associated with an increased risk of ASD. This can help researchers understand the genetic underpinnings of the disorder.
- Non-coding DNA Analysis: AI agents can analyze non-coding regions of the genome, which play a crucial role in gene regulation, to identify potential genetic variations contributing to ASD that might be missed by traditional analysis methods.
3. Modeling Brain Circuits and Connectivity:
- Network Analysis of the Brain: AI agents can be used to analyze brain connectivity data, revealing how different brain regions interact in individuals with ASD. This can help understand how disruptions in these neural circuits might contribute to the symptoms of the disorder.
- Simulating Brain Development: AI agents can be used to develop computational models that simulate healthy and abnormal brain development. These models can help researchers understand how genetic and environmental factors might influence the development of ASD.
4. Integrating Multimodal Data:
- Combining Data Sources: AI agents can integrate data from various sources, including brain imaging, genetics, and behavioral assessments, to create a more comprehensive picture of ASD. This holistic approach can lead to a deeper understanding of the disorder’s heterogeneity and potential subtypes.
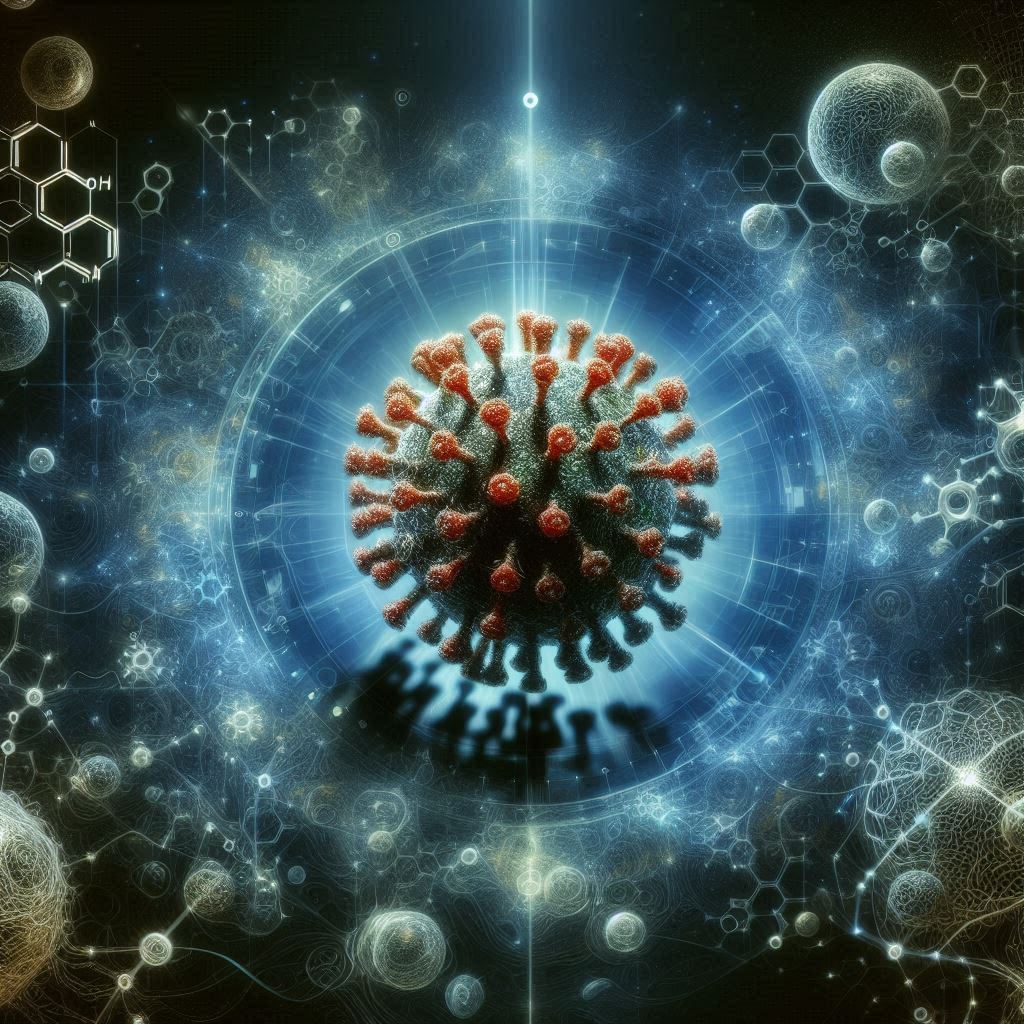
Infectious Diseases: What are the molecular mechanisms of emerging infectious diseases and how can we develop effective vaccines?
In the fight against emerging infectious diseases, AI agents are becoming valuable allies. Here’s how AI can help us understand the molecular mechanisms of these diseases and develop effective vaccines:
1. Analyzing Viral Genomes and Protein Structures:
- Genome Sequencing Analysis: AI agents can analyze the rapidly growing database of viral genomes from emerging pathogens. This allows identification of novel mutations, potential virulence factors, and similarities to existing viruses, providing insights into the origin and spread of the disease.
- Protein Structure Prediction: AI agents can predict the 3D structure of viral proteins, particularly those involved in host cell infection and immune response evasion. This knowledge is crucial for vaccine design, as it helps identify potential targets for antibodies.
2. Simulating Viral Evolution and Host Interactions:
- Predicting Mutations and Variants: AI agents can be used to model how viruses might mutate and evolve, allowing researchers to anticipate potential future strains and design broadly protective vaccines.
- Simulating Host-Pathogen Interactions: AI agents can simulate how emerging viruses interact with the human immune system, helping predict potential disease severity and identify weaknesses in the virus for vaccine development.
3. Accelerating Vaccine Design and Development:
- Reverse Vaccinology: AI agents can analyze viral genomes to identify potential vaccine targets, such as specific proteins or epitopes that trigger a strong immune response. This can significantly reduce the time and resources needed for traditional vaccine design methods.
- Vaccine Candidate Screening: AI agents can be used to rapidly screen large libraries of potential vaccine candidates, identifying the most promising ones for further development and clinical trials.
4. Outbreak Surveillance and Prediction:
- Real-Time Data Analysis: AI agents can analyze data from various sources, including genetic sequencing of viral samples, social media, and travel patterns, to identify and track outbreaks in real-time. This allows for earlier intervention and quicker development of targeted vaccines.
- Predicting Outbreaks: AI agents can be used to analyze historical data and identify factors that contribute to disease outbreaks. This knowledge can help develop predictive models and potentially prevent future outbreaks.
Infectious Diseases: How can we combat antimicrobial resistance globally?
In the ever-growing fight against antimicrobial resistance (AMR), AI agents are emerging as powerful allies. Here’s how AI can help us combat AMR globally:
1. Tracking and Monitoring Resistance Patterns:
- Analyzing Surveillance Data: AI agents can analyze vast amounts of data on antibiotic use, resistance patterns, and hospital-acquired infections. This allows for real-time identification of emerging resistance threats and informs public health interventions.
- Predicting Outbreaks: AI agents can be used to predict the potential spread of antibiotic-resistant bacteria in different regions, considering factors like antibiotic use patterns and population movement.
2. Optimizing Antibiotic Use and Stewardship:
- Clinical Decision Support Systems: AI agents can analyze patient data and recommend the most effective antibiotic for a specific infection, considering factors like patient allergies and local resistance patterns. This promotes antibiotic stewardship and reduces unnecessary use.
- Personalized Treatment Plans: AI agents can be used to develop personalized treatment plans that combine antibiotics with other therapies, potentially reducing reliance on antibiotics and minimizing selection pressure for resistant bacteria.
3. Accelerating Discovery and Development of New Antibiotics:
- Drug Repurposing: AI agents can analyze vast databases of existing drugs to identify potential candidates for repurposing as antibiotics. This can significantly reduce the time and cost of developing new antibiotics.
- Virtual Drug Discovery: AI agents can be used for virtual drug discovery, simulating how potential drugs interact with resistant bacteria at a molecular level. This allows for the identification of new drugs that can target novel mechanisms of resistance.
4. Surveillance and Detection of Outbreaks:
- Rapid Diagnostic Tools: AI agents can be used to develop rapid diagnostic tools that can identify not only the presence of an infection but also the specific antibiotic resistance profile of the bacteria involved. This allows for faster and more targeted treatment decisions.
- Hospital Outbreak Management: AI agents can be used to analyze data from hospital settings to identify potential outbreaks of resistant bacteria early on, allowing for quicker implementation of infection control measures.
There are many other questions in healthcare and medicine that need to be addressed by AI agents:
Clinical Medicine and Healthcare Delivery
1. Chronic Disease Management
– How can we improve adherence to treatment plans for chronic diseases such as diabetes and hypertension?
– What are the best strategies for preventing lifestyle-related diseases?
2. Mental Health
– How can we better understand the biological basis of mental health disorders and develop targeted treatments?
– What are the long-term effects of psychotherapies compared to pharmacological treatments?
3. Healthcare Quality and Safety
– How can we reduce medical errors and improve patient safety in healthcare settings?
– What are the most effective strategies for promoting evidence-based medicine in clinical practice?
4. Telemedicine and Digital Health
– How can telemedicine be integrated into mainstream healthcare to improve access and quality of care?
– What are the cybersecurity risks associated with the widespread adoption of digital health technologies?
Public Health and Global Health
5. Health Inequalities
– What are the root causes of healthcare disparities based on race, ethnicity, socioeconomic status, etc.?
– How can we achieve universal health coverage and equitable access to healthcare globally?
6. Health Policy and Economics
– What policies are most effective in controlling healthcare costs while maintaining quality of care?
– How can we address the social determinants of health to improve overall population health outcomes?
Ethical, Legal, and Social Implications
7. Ethical Issues in Medical Research
– How should research involving vulnerable populations (e.g., children, prisoners) be ethically conducted?
– What are the ethical implications of genetic engineering and gene editing technologies?
8. Patient Privacy and Data Security
– How can we balance the benefits of health data sharing for research with patient privacy concerns?
– What are the legal and ethical challenges of using AI and machine learning in clinical decision-making?
Emerging Technologies and Innovations
9. Artificial Intelligence in Healthcare
– How can AI be used to predict disease outbreaks and optimize healthcare resource allocation?
– What are the potential biases in AI algorithms used in medical diagnostics and treatment planning?
10. Regenerative Medicine and Tissue Engineering
– How can we scale up regenerative therapies to treat widespread conditions like organ failure?
– What are the long-term safety considerations of stem cell therapies?
Environmental and Occupational Health
11. Impact of Environmental Factors on Health
– What are the health effects of exposure to air pollution, chemicals, and climate change?
– How can urban planning contribute to promoting healthy lifestyles and reducing disease burden?
Educational and Training Needs
12. Medical Education and Training
– How can medical education better prepare physicians for the challenges of modern healthcare delivery?
– What are the best practices for interprofessional education to improve patient outcomes?
Research Methodology and Innovation
13. Clinical Trial Design and Implementation
– How can we improve the efficiency and inclusivity of clinical trials to speed up drug development?
– What are the best methods for incorporating patient-reported outcomes into clinical research?
Healthcare System Sustainability
14. Healthcare Workforce
– How can we address healthcare workforce shortages and improve retention rates?
– What are the impacts of physician burnout on patient care and how can it be mitigated?
Emerging Infectious Diseases and Pandemics
15. Preparedness and Response
– How can we improve global preparedness for future pandemics and epidemics?
– What are the barriers to equitable distribution of vaccines during global health emergencies?
Aging Population and Long-Term Care
16. Geriatric Medicine
– What are the best practices for managing multiple chronic conditions in older adults?
– How can we promote healthy aging and improve quality of life in the elderly population?
One mission of Centaurs Fabs is to develop AI Agents that can assist healthcare professionals to address these difficult but important questions.